Autonomous driving is getting better with every technological advance. But for driverless cars to become truly safe in traffic, they need a sensor system that recognises objects in the street – reliably and with no margin for error.
Christian Tschoban and Christian Dunkel from Fraunhofer IZM have teamed up for the KameRad project to develop a system that combines radars and cameras using Through-Glass-Vias (TGVs) on wafer technology for sensor integration. They met with RealIZM to discuss the challenges of the project and the hurdles yet to be overcome for sensor systems in autonomous driving.
What is the KameRad project all about?
Christian Tschoban: Autonomous driving has now reached the point where it is possible to drive on public roads without a human driver at the wheel. In 2016, the first cars drove from Los Angeles to Las Vegas for the auto show. That was a competition at the time. There were car manufacturers, like Mercedes, Audi, BMW and, I think, Tesla was also there. They drove their show cars fully autonomously from the West Coast to Las Vegas to be there for the event.
The horror scenario for all car manufacturers is simple: You are in busy urban traffic, and your car has to know what is happening all around it. People, other cars, and any other obstacles in the street have to be detected quickly. One scenario could be that a playing child suddenly runs into the street, and the car has to come to an emergency stop. There is no sensor technology in the world that could do this. Radar or similar systems cannot detect the danger. The question of liability is always there, and we don’t know how this could be solved. This means that autonomous vehicles will likely continue to cause accidents for the foreseeable future.
In our KameRad project, our goal was to build a sensor that monitors the environment on a permanent basis, that can detect what is happening, and then respond correctly to the situation. Different sensors are combined to feed their data into a powerful computing unit, which processes the environment data. The system would need to be integrated well and easily installed in the car, so we applied high-integration technology. We have what is called target levels, which indicate when it would be possible to drive autonomously. These indicate the different points at which the car can take over and the human driver can sit back and enjoy the trip to the destination. Our intention is to have a sensor ready for level 4 or higher. This means that it should work both in out-of-town and in inner-city environments. We managed to do this by building a universal sensor platform that combines a stereo camera with a radar sensor in the car, around the engine. We also built a redundant sensor platform that connects the hardware and software. The thinking was to use this for decentralised computing, i.e. this computer unit has several computing cores and distributes its computing power across several platforms. Everything should be highly integrated, so that it fits together perfectly. We made a metal case for the system, because the specifications demanded that the sensor can be mounted inside and outside the car. This also means that the housing must be waterproof, because the sensor could be affected by heavy rain. The eventual size of the housing was determined by the stereo cameras and by the need to have the stereo cameras set at a certain distance from each other. They work like our human eyes, which are also set apart from each other. This is the essential principle of a stereo camera: It enables spatial vision.
To get to the interposer in our design, we built modules that are always the same. This means that everything that does complex high-frequency work should be ready for mass production with high-frequency-ready production facilities. For our interposers, we used glass as the base material. We compared silicon and glass in our tests and found that antennas work better on glass. That is why we chose it. Christian Dunkel made the small glass plates the radar front sits on.
How were the requirements for the system defined at the beginning of your development work?
Christian Tschoban: At the beginning, we charted a virtual route in Berlin, from Zoologischer Garten, or specifically, from the VDE/VDI offices, to the Federal Ministry of Education and Research, which had financed this amazing project. It has roundabouts, double carriageways, cars overtaking each other, and everything else that goes with it. We then played back this route virtually and defined use cases from it. Which use cases could occur in this area? And then we set up large Excel tables and took out the worst-case scenarios for our radar and camera system. That’s how we proceeded.
Are we only talking about the automotive industry, or could such systems also be used for trains or ships?
Christian Tschoban: These systems are designed for the automotive industry, which means that this system is only meant to cover a directional range in the horizontal plane. With ships and aircraft, however, it would be necessary to include the vertical plane as well. This is not possible with the KameRad system. This is a weakness of all radar systems used in the automotive industry, because it is always assumed that driving is only done on a level surface. If a car e.g. goes into a tunnel which has its entrance at an angle, it could happen that the radar system’s alarms go off. The car industry solves this problem by simply turning off the short-range radar above a certain speed, usually 30 kilometres per hour.
Why did you decide to use a combination of radar and camera modules and not another sensor system like LIDAR?
Christian Tschoban: Let me quickly recap what LIDAR is to explain our decision. LIDAR is a high-resolution method, in which a light signal is emitted and picked up again as an electromagnetic wave. The frequency range differs from radar, but the principle is the same. The advantage of LIDAR is the extremely high resolution that is possible because light can cover incredibly large areas. The disadvantage of LIDAR systems is that they cannot do their detecting work through fog or rain, whereas radar systems have no problems with moisture. Camera systems have the same drawback as LIDAR, but they are already commonplace in cars, which is why we decided to use them. In addition, we had a partner on the project consortium, Fraunhofer FOKUS, who are specialised in camera systems and in combining cameras with other sensors. That’s why we came up with the idea of combining cameras and radar. Of course, you could also combine cameras and LIDAR or radar and LIDAR, and there are other research projects that do exactly that.
LIDAR is much more expensive than radar, though. Radar systems cost less than 100 euros, whereas today’s LIDAR systems come in around 500 to 1000 euros. But LIDAR is better by a factor of ten in terms of resolution. The two worlds are always competing with each other. Attempts are currently being made to make LIDAR systems cheaper, while the other side is trying to increase the resolution of radar systems. LIDAR or radar are getting more popular in the automotive industry every year, but the received opinion is that autonomous driving will always require a combination of different sensors.
Is the system also capable of learning, or are we only talking about sensor systems here?
Christian Tschoban: The system cannot learn as such, because that was not our goal. The goal was rather to build a platform. Learning is now coming into focus for the follow-up project called AI Radar. In this project, the cameras are actually now being replaced by LIDAR. But this is an external LIDAR system.
What exactly did Fraunhofer IZM contribute to the KameRad project?
Christian Tschoban: We developed the radar, the cameras, and also the interposer. And we worked with InnoSenT GmbH to design the circuit diagram. The layout and placement of the components was also done by us. The firmware was provided by:
- AVL Software and Functions GmbH,
- InnoSenT GmbH,
- John Deere GmbH,
- Jabil Optics Germany GmbH,
- Silicon Radar,
- TU Berlin (DCAITI),
- Fraunhofer FOKUS.
What makes this system more competitive than those from other suppliers?
Christian Tschoban: Car manufacturers are not radar manufacturers. Essentially, they buy metal boxes that they screw onto or into their cars. There are others, and they have similar solutions to ours, some of which may honestly even be better. But our idea was to focus on the complex pieces, for which you need a quite a lot of special know-how, for a mass-production process. The end product is an exact structure with low tolerances, made reliably in a wafer-level process. In the end, you only need to solder on these modules. The trick with us is that you can change the resolution of the radar system by changing the number of modules. For example, the system has a resolution of 12.5 degrees, but if two modules are used, you get up to six degrees, and four modules mean a resolution of less than two degrees. This gives us a competitive edge compared with designs that combine everything on a single PCB. The basic idea behind this is simple: I have a modular kit that lets me build my radar system in a way that fits the application perfectly. Another difference compared to other suppliers is that they only produce systems that are integrated behind the windscreen. Our system can be mounted on the outside as well.
Why did you choose glass as a material? How do you benefit from that?
Christian Tschoban: The big advantage of glass is that you can really work it in wafer-level processes. That is exactly what Christian Dunkel did with his colleagues at Fraunhofer IZM-ASSID. Glass as a material has good high-frequency properties, and it lets you cut structures with extremely low tolerances. Moreover, it can be pushed through in interposer production. Christian Dunkel did not produce individual parts, but complete wafers with separate structures. You get very good reproducibility, of course, which makes it suitable for mass production. So, in sum, the advantage relates to interposer production and to the opportunity to scale it to mass production.
Christian Dunkel: It is manufactured with the right equipment, which we have here. This means that 16 chips can be put on a 200 millimeter wafer, and twice as many chips can be produced on a 300 millimetre wafer.
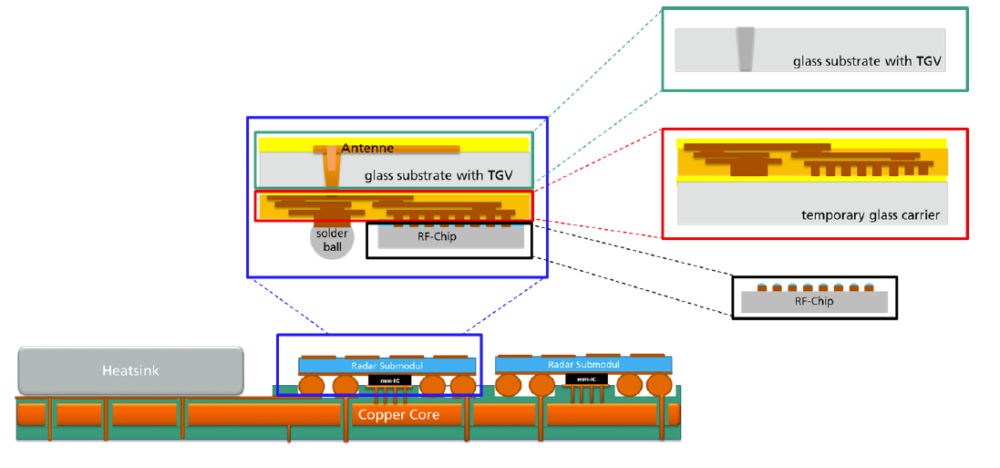
And why did you choose the TGV (Through-Glass-Via) technology?
Christian Tschoban: We chose TGVs because the chip at the bottom has to be connected with the antennas at the top. That’s what the TGVs are needed for. Our colleagues also wanted to develop TGVs for the project; that is one of the innovations: that you can see TGVs on the wafer. And it was also one of the challenges Christian Dunkel had to deal with: How is it possible to reliably produce TGVs on the wafer scale?
Christian Dunkel: The main problem we had with this project was the following: There is a hole through the wafer, which we have to be able to handle with our equipment. Many systems have the problem that, as soon as there is a hole in the wafer, the contact ring is soiled during plating, or the electrolyte comes through to the back of the wafer. We solved this by first producing the back of the wafer on a carrier wafer. Then we created a transfer bond on the TGV wafer, which closed the TGV down to the RDL. This allowed us to process the wafer in the normal way, just like any other wafer.
To what extent did size, computing power, and power consumption influence the design of the sensor system?
Christian Tschoban: In the beginning, power consumption was not our focus. At that time, we were still working on the basis of a petrol engine, and with a petrol engine, you do not have a power problem. Of course, this is changing with electric cars, especially for systems that are used permanently. This means, for example, urban scenarios in which the radar system should continue to run even when the car is stationary. This would also affect internal combustion engines, because such a scenario would naturally put a strain on their batteries. The challenge with electric cars is that the cars are set up in such a way that they can cover approx. 300 to 400 km with power consumption as it is now. But if power consumption gets much higher, due to radar systems of this kind, the cars will start to reach their limits. As I said, this was not originally a concern for us, but it is now.
Concerning integration, the size of the module was determined by the stereo cameras. But size requirements are actually influenced much more by cost considerations. Space on a wafer costs money, and the smaller we can make the modules, the more money we can save. In principle, however, antenna spacing now determines the size of the module. Therefore, the goal was to make the system as small as possible and with maximum system apparatus. That is how the structural size came about. We had two opposing goals for optimizing our design: as large as possible for good resolution, and as small as possible to keep costs down.
Is it a fail-safe sensor system? If it is, how can you make sure that the system will always work without fail?
Christian Tschoban: The basic idea behind it is that several sensors are combined, so you can guarantee greater reliability; i.e. that the sensor actually detects what is in front of it. The combination of camera and radar should therefore be able to detect any disturbance. We cannot say whether absolute reliability is given, because we have not done the tests yet. What we can say, however, is that all the conditions we had in the use cases were met. Fail-safe means that everything is always fault-free in all situations. To achieve that, the software, the hardware, and everything else would have to function reliably for 10 years. However, the combination of these two sensors can already cover many standard situations, such as fog, entering a tunnel, or rain.
Christian Dunkel: What we produced was a prototype to demonstrate the feasibility of the concept. For its actual application in cars, we would need further testing and development in order to achieve such a reliable state.
If we look at the real application of the system, could it recognise whether something is just a leaf falling from a tree or actually a child running into the street, or would the car brake in both cases?
Christian Tschoban: The system can actually detect quite a lot. But there was one scenario that gave everyone a bit of a headache. Imagine a can of coke on the road. It has the same radar cross-section as a human being. This means that if you drive up to a can of coke, today’s radar systems would cause the car to brake hard. We even simulated this, and in our test, the radar system was able to recognise quite well that it was not a human being. The cameras then confirmed that it was not a person. Our colleagues at Fraunhofer FOKUS even used machine learning to teach the system to distinguish leaves on the road or to recognise whether an obstacle is a pedestrian or not.
About real-time communication: Will the system be compatible with 5G or 6G in the future?
Christian Tschoban: The system has an LTE, KTX interface. KTX is a separate standard that can be upgraded to 5G. It is a high-end PC with two graphics cards and two processors, so it is like a server. The system even has a USB drive and an internet connection.
What were the biggest challenges in this project for you?
Christian Dunkel: For me, the biggest challenge was definitely the integration of the TGV wafer according to the specifications of the project. That was something we had never done before. It took quite a bit of learning until we could send a module to Christian Tschoban that was how he wanted it. This was partly because we do not produce the TGVs ourselves. The companies making them also encountered challenges, for example with chipping. Their first versions had small cracks at the inputs and outputs of the wafers. Of course, this is very difficult to coat and certainly not conducive to reliability. Other suppliers then used other processes, which did not cause any cracks. As I said, that alone was a learning process.
Christian Tschoban: We took care of the entire module design, so communication with all partners was our responsibility. Since the individual module designs were complex, for example the stereo cameras, but also the radar system, it was challenging to coordinate this process again and again with all partners. Nevertheless, cooperating with the colleagues was a lot of fun.
Do you already have follow-up projects? What are your future prospects?
Christian Tschoban: We already started a follow-up project in 2020. It is no longer so much about building the interposer, but more about system integration. In the new project, we are working on a different technology to bring the frontend things closer together with the processing and to move more in the direction of AI. We are also planning another follow-up project. There is currently a new BMBF tender for the OCTOPUS project, and we are trying to set this up with the same consortium. The idea behind this is to bring more computing power into the system, so that machine learning becomes even better. There will also be greater emphasis on security.
The Interview was conducted and edited by Jacqueline Kamp on June 9, 2021
Picture: Fraunhofer IZM / Volker Mai
Add comment